Traffic modeling
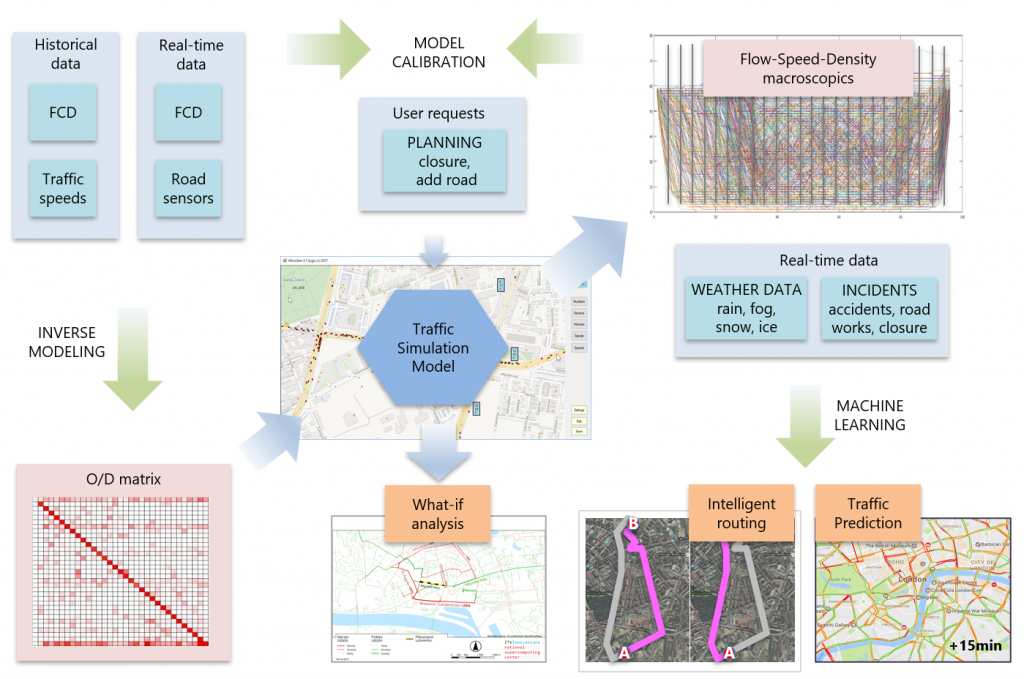
In Europe, urban traffic congestion costs the EU nearly 1 percent of its annual GDP and accounts for 40 percent of all CO2 emissions. Statistically, driving in congested conditions increases CO2 emission by 80%. Travel times in mega cities during rush hours are on average increased by 50 to more than 100%. And yet, the concentration of people in cities continues to grow. In Europe the percentage of citizens living in urban areas will rise from 73% in 2010 to 80% in 2050. To evaluate the quality of the transport infrastructure, traffic models are used to characterize the road network by quantification of the following macroscopic parameters: traffic flow, density, and average speed (so called 3D traffic model), ideally for all road segments and for each time interval.
In this project the framework of traffic modeling is extended to a calculate precise 3D traffic model represented by the macroscopic tuple (flow, speed, density) obtained for all road elements at given time windows and keep it constantly up to date with an extensive use of machine learning. This requires processing of several data sources such as historical and real-time floating car data (FCD) of moving vehicles and weather data (precipitation, temperature). Once the solid traffic model is available, it will be used for subsequent computation of traffic prediction and truly intelligent routing for global traffic optimization flow using various machine learning techniques.